Written by: Iyan E. Mulia
A chapter by Dr. Iyan E. Mulia, a Hydrography Research Group member, has been published in a book entitled “Probabilistic Tsunami Hazard and Risk Analysis: Towards Disaster Risk Reduction and Resilience” (https://doi.org/10.1016/C2022-0-00360-3). The book comprises a collection of articles from tsunami experts globally. The chapter discusses the application of machine learning (ML) in tsunami forecasting and early warning systems, emphasizing its potential to enhance the accuracy and efficiency of predictions. In the context of hydrography—the study of the physical features of bodies of water and the adjacent land areas—the chapter is relevant in several aspects, such as data collection and analysis, modeling, and impact on coastal management.
Hydrography involves collecting data related to water bodies, including sea level, wave heights, and ocean currents. The chapter highlights various ocean observation networks, such as the Seafloor Observation Network for Earthquakes and Tsunamis (S-net), which provide critical data for tsunami forecasting models. This data is essential for ML algorithms to analyze and predict tsunami behavior effectively. Consequently, accurate data analysis is an integral part of the ML model that should be considered before developing the tsunami forecasting system. Integrating hydrographic data with other data types, such as seismic and geodetic data, is also essential to compensate for the scarcity of tsunami records. The versatility of ML allows for such data fusion to improve prediction accuracy.
The chapter also discusses how ML models can be trained using data from hydrographic observations to forecast tsunami waveforms and inundation patterns. This modeling is crucial for understanding how tsunamis interact with coastal environments, a key aspect of hydrography. The new modeling paradigm of ML offers several advantages over conventional models. For instance, ML models have a remarkable computing speed compared to the traditional tsunami model and thus are suitable for early warning. Furthermore, ML-tsunami models typically have lower memory and data storage, making them more efficient for modern computational infrastructures like cloud and edge computing. The integration of ML with Internet of Things (IoT) sensors is also explored, suggesting that this combination could significantly improve tsunami early warning systems. However, the “black-box” nature of ML often complicates model interpretability. This issue can undermine the reliability of predictions, especially when using complex architectures.
The insights gained from ML applications in tsunami forecasting can inform coastal management strategies. By understanding potential inundation areas and wave heights, particularly in real-time, authorities can develop better evacuation plans and infrastructure resilience, which are essential components of hydrographic studies. In summary, the chapter illustrates how advancements in ML, supported by hydrographic data, can significantly improve tsunami early warning systems, ultimately contributing to better disaster preparedness and response.
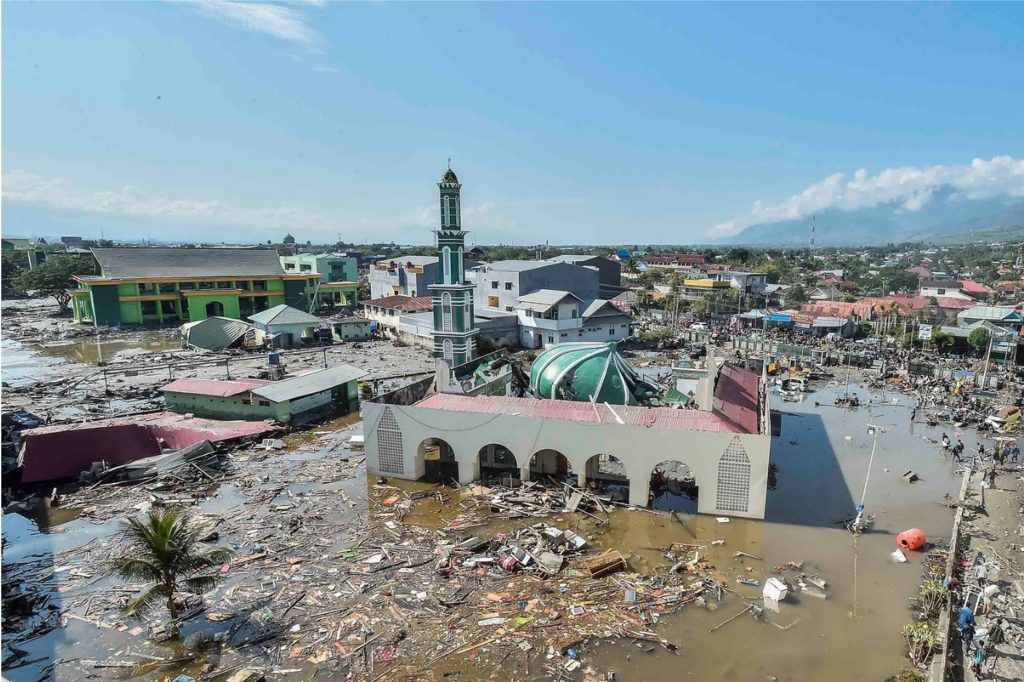
Illustration of tsunami impact (Palu, 30 September 2018). Source: Muhammad Adimaja / Antara Foto Agency / Reuters.